Model that maps relationships between neurons could help make deep brain stimulation treatment more viable
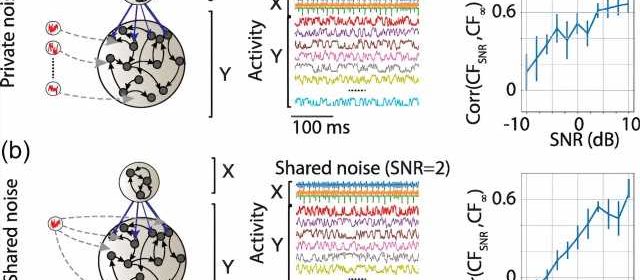
By listening in on the background chatter in the brain, scientists can predict the relationships between different neurons, according to new research from University of Oregon scientists and their colleagues.
The advance could help make deep brain stimulation a more viable treatment for conditions like Parkinson’s, epilepsy and depression, allowing doctors to figure out exactly which neurons to stimulate without extensive trial and error.
The findings are reported in a paper published Dec. 7 in the journal Physical Review Research.
Deep brain stimulation is an emerging treatment for certain neurological and psychiatric conditions that haven’t responded to other treatments. Electrodes are surgically implanted into the brain so that they stimulate specific neural circuits, alleviating symptoms.
But effectively using those electrodes is difficult. Each one contains many different channels that can stimulate different spots, and even neurons right next to each other can have wildly different roles. Finding the right spot to stimulate involves a lot of trial and error, which is both costly and risky.
“What we thought about in this paper is, instead of doing trial and error, maybe we could predict the effect of the stimulation before we stimulate,” said Luca Mazzucato, a computational neuroscientist in the UO’s College of Arts and Sciences who co-led the work alongside Roozbeh Kiani at New York University.
The human brain contains roughly 100 billion neurons, so understanding the relationships between them is far from straightforward. When so many variables are at play, it can be especially challenging to figure out when one neuron is causing the activity of another neuron downstream, versus some other outside force affecting both of them in tandem.
To untangle this problem within the complexity of the brain, Mazzucato found inspiration in the field of ecology. He riffed off of a set of mathematical equations originally developed by biologist George Sugihara at the Scripps Institute to understand how fluctuating ocean temperatures were affecting fish populations. Those equations are designed to untangle cause-and-effect relationships between different variables that might seem unlinked on the surface.
Mazzucato and his colleagues found they could use the same methods in the brain, to predict relationships between certain brain circuits by looking for patterns in the spontaneous background activity of neurons.
The NYU researchers recorded brain activity from the prefrontal cortex in macaque monkeys, to get readings of background activity. Then, Mazzucato and his team used the equations to predict causal relationships between different neurons. They could test their predictions by stimulating certain brain areas and seeing which neurons responded.
Their mathematical modeling worked, the team found. It accurately mapped relationships between neurons and allowed scientists to predict the effects of stimulating in certain places.
The finding opens up exciting avenues for future experimentation. The researchers next hope to test whether these causal links between neurons are related to animal behavior—that is, whether it’s possible to predict how stimulating a certain part of the brain will affect behavior.
Eventually, team members hope their research will lead to improved treatments for complex neurological conditions, via deep brain stimulation or even brain-machine interfaces that allow two-way communication between a brain and a computer.
More information:
Amin Nejatbakhsh et al, Predicting the effect of micro-stimulation on macaque prefrontal activity based on spontaneous circuit dynamics, Physical Review Research (2023). DOI: 10.1103/PhysRevResearch.5.043211
Journal information:
Physical Review Research
Source: Read Full Article