Mathematical Modeling of Cell Cycle Dynamics

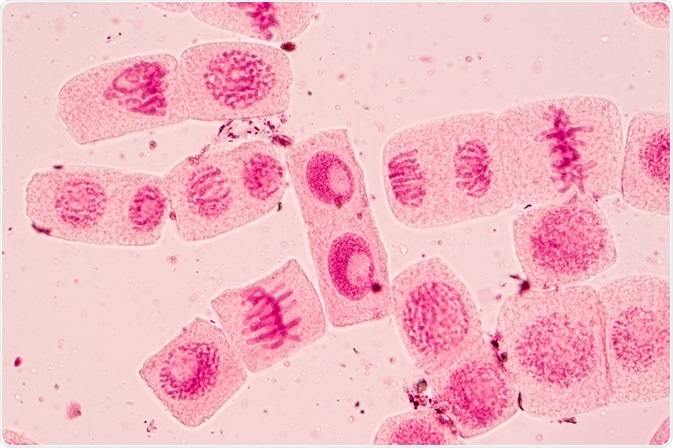
Why do we need to understand the dynamics of the cell cycle?
The cell cycle is a mechanism that controls and integrates the stages of DNA synthesis, mitosis, and cell division. This mechanism dictates growth and development, as well as reproduction. Therefore, gaining a thorough understanding of the cell cycle at the molecular level is vital to understanding not only humans but all eukaryotes. Establishing a comprehensive knowledge of the cell cycle is one of the most sought-after goals of the field of cell biology.
As biologists uncover more about the complex network of cell cycle controls, scientists are finding it increasingly challenging to predict how amending on one component of the cycle will impact the system overall. However, these predictions are essential for gaining an understanding of how deviations from the normal cell cycle lead to disease. This knowledge could be vitally important in deepening our knowledge of cancer as well as developing effective new therapeutics and preventative strategies.
Using mathematical modeling to understand the cell cycle
While mathematical modeling is not considered to be a traditional experimental method, it is a tool with the potential to uncover fine-grain detail of the complex cell signaling networks involved in managing the eukaryotic cell division cycle.
Mathematical models are capable of organizing large datasets obtained from experimental studies. They have been demonstrated to be capable of using this experimental data to describe the fundamentals of the cell cycle system as well as filling in the gaps where data has failed to provide answers. Mathematical models are incredibly useful for driving hypothesis development, helping to guide scientists to explore routes that have the most potential.
Already, distinct scientific fields, such as chemical engineering and meteorology, have demonstrated the benefit of using mathematical modeling to uncover the fundamental behaviors of complex systems. Until recently, however, mathematical modeling had been under-appreciated in the field of molecular biology. Fortunately, recent years have seen scientists working in this discipline of mathematical modeling to reveal key insights about cell-cycle dynamics.
Uncovering oscillating growth patterns
The proliferation of cells is vital to ensuring normal biological processes, it also underlies pathological processes. Since mathematical models were first given attention by molecular biology, numerous models of proliferation have been established. However, these models often assume that cell proliferation is exponential. However, cells that are in the same cell cycle phase are synchronous, or partially synchronous if a subpopulation of the cells is considered synchronous.
In the case of synchronous cells, division happens in discrete stages, and thus the growth rate produced is variable per capita, not exponential. Therefore, models that have already been established to describe cell cycle dynamics do not account for subpopulations and predict exponential growth that is not always the case.
A 2019 study provided new experimental data collected from two-dimensional cell proliferation assays where observed cell growth was deemed to appear exponential. The team was able to prove that oscillatory subpopulations arose from what the researchers referred to as inherent synchronization. The study employed fluorescent ubiquitination-based cell cycle indicator (FUCCI) to identify subpopulations based on the cell cycle phase, revealing the inherent synchronization that is usually hidden.
This data is key, it shows that while cell populations may seem to grow exponentially, they may contain subpopulations that exhibit oscillatory growth due to inherent synchronization.
This research highlights the fact that previous mathematical modeling of cell cycles did not capture the whole picture as they did not account for subpopulations with oscillating growth. The new model established through this research will likely be useful for understanding mechanisms that are dependent on the cell cycle, like responses to therapeutics, for example.
Using mathematical modeling to reveal cell cycle dynamics of tumors
More recent research has used mathematical modeling to reveal key characteristics of the cell cycle of tumors, which may lead to more effective cancer therapeutics.
In oncology, tumor spheroids are commonly used as an in vitro assay for investigating how different cancer cell lines respond to therapeutics. They have become popular mostly because of how spheroids grow, with the tumor cells consuming the oxygen and nutrients within the culture medium, causing localized cell proliferation at the boundary of the spheroid.
Cells at the spheroid’s center become hypoxic as the spheroid grows and eventually dies, creating a necrotic core. The localized proliferation of tumor cells at the surface creates pressure that generates a cellular flow of tumor cells originating at the tumor’s edge and moving towards the core. Studies have shown that this flow allows inert microspheres to enter the tumor spheroids at the surface.
In a 2020 study, scientists re-assessed this model using an off-lattice hybrid agent-based model and attempted to define to what extent these microspheres could be used to describe the kinetic parameters associated with the tumor spheroids.
The researchers found that differing rates of tumor cell proliferation and hypoxia sensitivity can create spheroids that have similar bulk growth dynamics but very different compositions internally. The team used their evidence to produce a model that could be used to infer spheroid composition and parameters related to tumor cell lines and highlight the inefficacy of previous models that relied on calculating individual microbead trajectories.
Sources
- Bull, J., Mech, F., Quaiser, T., Waters, S. and Byrne, H., 2020. Mathematical modelling reveals cellular dynamics within tumour spheroids. PLOS Computational Biology, 16(8), p.e1007961. journals.plos.org/ploscompbiol/article?id=10.1371/journal.pcbi.1007961
- Sible, J. and Tyson, J., 2007. Mathematical modeling as a tool for investigating cell cycle control networks. Methods, 41(2), pp.238-247. https://www.ncbi.nlm.nih.gov/pmc/articles/PMC1993813/
- Vittadello, S., McCue, S., Gunasingh, G., Haass, N. and Simpson, M., 2019. Mathematical models incorporating a multi-stage cell cycle replicate normally-hidden inherent synchronization in cell proliferation. Journal of The Royal Society Interface, 16(157), p.20190382. https://royalsocietypublishing.org/doi/10.1098/rsif.2019.0382
Further Reading
- All Cell Cycle Content
- The Cell Cycle
- What Are Cyclins?
- Cell-Cycle-Dependent Regulation of Cell Adhesions
Last Updated: Jun 2, 2021
Written by
Sarah Moore
After studying Psychology and then Neuroscience, Sarah quickly found her enjoyment for researching and writing research papers; turning to a passion to connect ideas with people through writing.
Source: Read Full Article